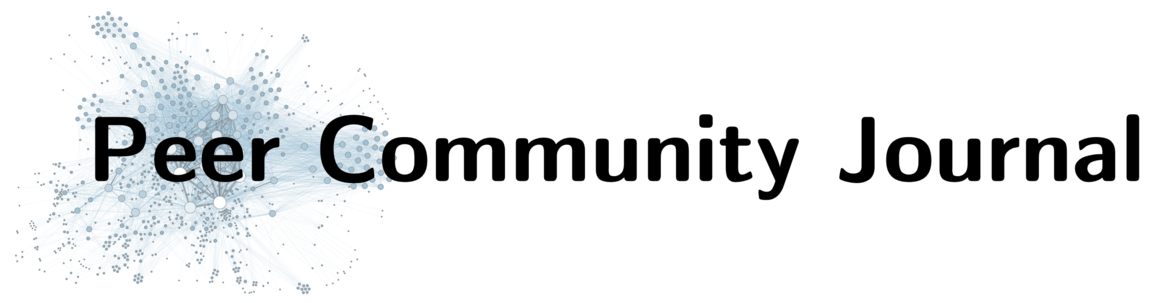
Latest Articles
-
Section: Animal Science ; Topics: Agricultural sciences
Goats who stare at video screens – assessing behavioural responses of goats towards images of familiar and unfamiliar con- and heterospecifics
10.24072/pcjournal.473 - Peer Community Journal, Volume 4 (2024), article no. e94.
Get full text PDFMany cognitive paradigms rely on active decision-making, creating participation biases (e.g. subjects may lack motivation to participate in the training) and once-learned contingencies may bias the outcomes of subsequent similar tests. We here present a looking time approach to study goat perception and cognition, without the need to extensively train animals and no reliance on learned contingencies. In our looking time paradigm, we assessed the attention of 10 female dwarf goats (Capra hircus) towards 2D visual stimuli which were images of familiar and unfamiliar con- and heterospecifics (i.e. goats and humans) using an experimental apparatus containing two video screens. Spontaneous behavioural reactions to the presented stimuli, including the looking behaviour and the time spent with the ears in different positions were analysed using linear mixed-effects models. We found that goats looked longer at the video screen presenting a stimulus compared to the screen that remained white. Goats looked longer at images depicting other goats compared to humans, while their looking behaviour did not significantly differ when being confronted with familiar vs. unfamiliar individuals. We did not find statistical support for an association between the ear positions and the presented stimuli. Our findings indicate that goats are capable of discriminating between two-dimensional con- and heterospecific faces, but also raise questions on their ability to categorise other individuals regarding their familiarity using 2D face images alone. Our subjects might either lack this ability or might be unable to spontaneously recognise the provided 2D images as representations of real-life subjects. Alternatively, subjects might have shown an equal amount of motivation to pay close attention to both familiar and unfamiliar faces masking potential effects. The looking time paradigm developed in this study appears to be a promising approach to investigate a variety of other research questions linked to how domestic ungulate species perceive their physical and social environment.
-
Section: Ecology ; Topics: Biophysics and computational biology, Ecology
Predicting species distributions in the open ocean with convolutional neural networks
10.24072/pcjournal.471 - Peer Community Journal, Volume 4 (2024), article no. e93.
Get full text PDFAs biodiversity plummets due to anthropogenic disturbances, the conservation of oceanic species is made harder by limited knowledge of their distributions and migrations. Indeed, tracking species distributions in the open ocean is particularly challenging due to the scarcity of observations and the complex and variable nature of the ocean system. In this study, we propose a new method that leverages deep learning, specifically convolutional neural networks (CNNs), to capture spatial features of environmental variables. This novelty eliminates the need to predefine these features before modelling and creates opportunities to discover unexpected correlations. Our aim is to present the results of the first trial of this method in the open ocean, discuss limitations and provide feedback for future improvements or adjustments. In this case study, we considered 38 taxa comprising pelagic fishes, elasmobranchs, marine mammals, marine turtles and birds. We trained a model to predict probabilities from the environmental conditions at any specific point in space and time, using species occurrence data from the Global Biodiversity Information Facility (GBIF) and environmental data from various sources. These variables included sea surface temperature, chlorophyll concentration, salinity and fifteen others. During the testing phase, the model was applied to environmental data at locations where species occurrences were recorded. The classifier accurately predicted the observed taxon as the most likely taxon in 69% of cases and included the observed taxon among the top three most likely predictions in 89% of cases. These findings show the adequacy of deep learning for species distribution modelling in the open ocean. Additionally, this purely correlative model was then analysed with explicability tools to understand which variables had an influence on the model’s predictions. While variable importance was species-dependent, we identified finite-size Lyapunov exponents (FSLEs), sea surface temperature, pH and salinity as the most influential variables, in that order. These insights can prove valuable for future species-specific ecology studies.
-
Section: Animal Science ; Topics: Genetics/genomics
Cost-efficient assignment panel for ducks. Setup of a cost-efficient assignment panel for duck populations.
10.24072/pcjournal.475 - Peer Community Journal, Volume 4 (2024), article no. e92.
Get full text PDFThe setup of a flexible and cost-effective 96-SNP assignment panel to be used in Pekin duck (Anas platyrhynchos), Muscovy duck (Cairina moschata) and their mule duck hybrid, is presented. SNP were selected on the available 600K array in ducks. This SNP array is made of two libraries (one for the Muscovy duck, the other for the common duck which encompasses the Pekin duck), the intersection of which, after a preliminary elimination on the primer length, contained only 399 SNP that were considered a starting point to obtain a final list. A first step was to obtain a list of 192 SNP, based on technical properties, using a reference set of 600K genotypes from commercial lines. In a second step, to obtain the final 96 markers, a subset of the previous reference set was combined with genotypes from 133 Pekin and 127 Muscovy, which were the parents of the experimental populations to assign. Assignment rates were 99%, 96% and 88% in the mule, Pekin and Muscovy populations respectively. The lower-than-expected assignment rate in the Muscovy population was due to the absence of 16 parental samples. Availability of an effective and affordable assignment panel was deemed necessary after switching from a system where breeders are housed in individual cages to a system where females are housed and inseminated in groups. In the latter case, a factorial mating design replaced the hierarchical design, common in poultry. This new design impacted the population structure, creating more sire x dam combinations, offering possibilities for a better estimation of non-additive genetic effects, which could prove relevant in the foie gras sector. Finally, a list of 135 markers resulted from this study that could be used to build an efficient 96 SNP panel for any local or commercial population.
-
Section: Genomics ; Topics: Genetics/genomics, Microbiology
A Comprehensive Resource for Exploring Antiphage Defense: DefenseFinder Webservice,Wiki and Databases
10.24072/pcjournal.470 - Peer Community Journal, Volume 4 (2024), article no. e91.
Get full text PDFIn recent years, a vast number of novel antiphage defense mechanisms were uncovered. To facilitate the exploration of mechanistic, ecological, and evolutionary aspects related to antiphage defense systems, we released DefenseFinder in 2021 (Tesson et al., 2022). DefenseFinder is a bioinformatic program designed for the systematic identification of known antiphage defense mechanisms. The initial release of DefenseFinder v1.0.0 included 60 systems. Over the past three years, the number of antiphage systems incorporated into DefenseFinder has grown to 152. The increasing number of known systems makes it a challenge to enter the field and makes the interpretation of detections of antiphage systems difficult. Moreover, the rapid development of sequence-based predictions of structures offers novel possibilities of analysis and should be easily available. To overcome these challenges, we present a hub of resources on defense systems, including: 1) an updated version of DefenseFinder with a web-service search function, 2) a community-curated repository of knowledge on the systems, and 3) precomputed databases, which include annotations done on RefSeq genomes and structure predictions generated by AlphaFold. These pages can be freely accessed for users as a starting point on their journey to better understand a given system. We anticipate that these resources will foster the use of bioinformatics in the study of antiphage systems and will serve the community of researchers who study antiphage systems. This resource is available at: https://defensefinder.mdmlab.fr.
Follow us
People
Sections
- Animal Science 20
- Archaeology 20
- Ecology 89
- Ecotoxicology & Environmental Chemistry 8
- Evolutionary Biology 83
- Forest & Wood Sciences 7
- Genomics 37
- Health & Movement Sciences 3
- Infections 28
- Mathematical & Computational Biology 17
- Microbiology 12
- Network Science 5
- Neuroscience 7
- Organization Studies 1
- Paleontology 10
- Registered Reports 1
- Zoology 22
Conferences
Indexed by
Supporters
Membership
Image Credits
The network image was drawn by Martin Grandjean: A force-based network visualization CC BY-SA